Artificial Intelligence (AI) has become an integral part of modern society, influencing various sectors such as healthcare, finance, transportation, and entertainment. However, the rapid advancement and widespread adoption of AI technologies have raised numerous ethical challenges. These challenges are multifaceted and complex, requiring careful consideration and proactive measures to ensure that AI systems are developed and deployed responsibly. Below, I will outline and discuss in detail the major ethical challenges in AI, using a structured approach to clearly distinguish the contents of each major paragraph. |
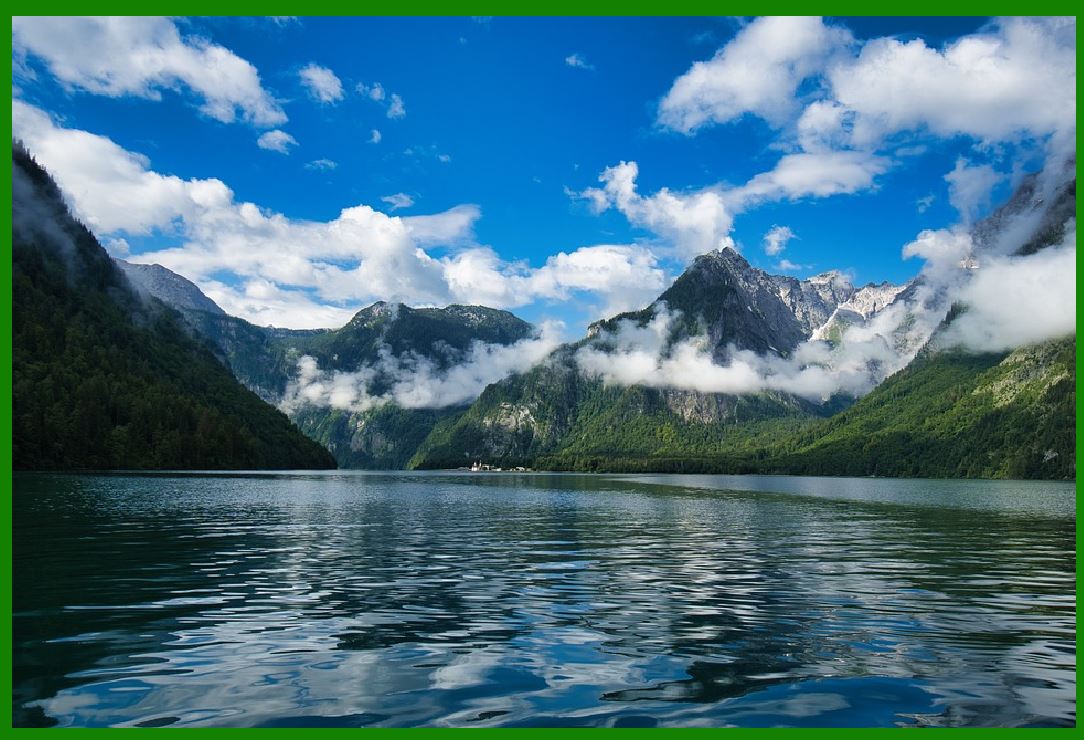
|
1. Bias and Discrimination |
One of the most significant ethical challenges in AI is bias and discrimination. AI systems are trained on large datasets that often contain historical biases and prejudices. When these biases are not adequately addressed, AI systems can perpetuate and even amplify discriminatory practices. This issue is particularly concerning in areas such as hiring, lending, law enforcement, and healthcare, where biased AI systems can lead to unfair treatment of individuals based on race, gender, age, or other protected characteristics. |
1.1 Sources of Bias |
Bias in AI can originate from various sources, including: |
Training Data: If the data used to train AI models is biased, the resulting AI system will likely exhibit similar biases. For example, if a hiring algorithm is trained on data that reflects historical gender biases, it may favor male candidates over equally qualified female candidates. |
Algorithm Design: The design of AI algorithms can also introduce bias. For instance, certain algorithms may prioritize specific features that inadvertently disadvantage certain groups. |
Human Intervention: Bias can be introduced through human intervention during the development and deployment of AI systems. Developers?own biases and assumptions can influence the behavior of AI systems. |
1.2 Mitigation Strategies |
To address bias and discrimination in AI, several strategies can be employed: |
Diverse and Representative Data: Ensuring that training data is diverse and representative of the population can help reduce bias. This involves collecting data from various sources and ensuring that all relevant groups are adequately represented. |
Bias Detection and Mitigation: Implementing techniques to detect and mitigate bias in AI systems is crucial. This can include using fairness-aware algorithms, conducting regular audits, and employing bias detection tools. |
Transparency and Accountability: Promoting transparency and accountability in AI development can help address bias. This involves making AI systems?decision-making processes more transparent and holding developers accountable for biased outcomes. |
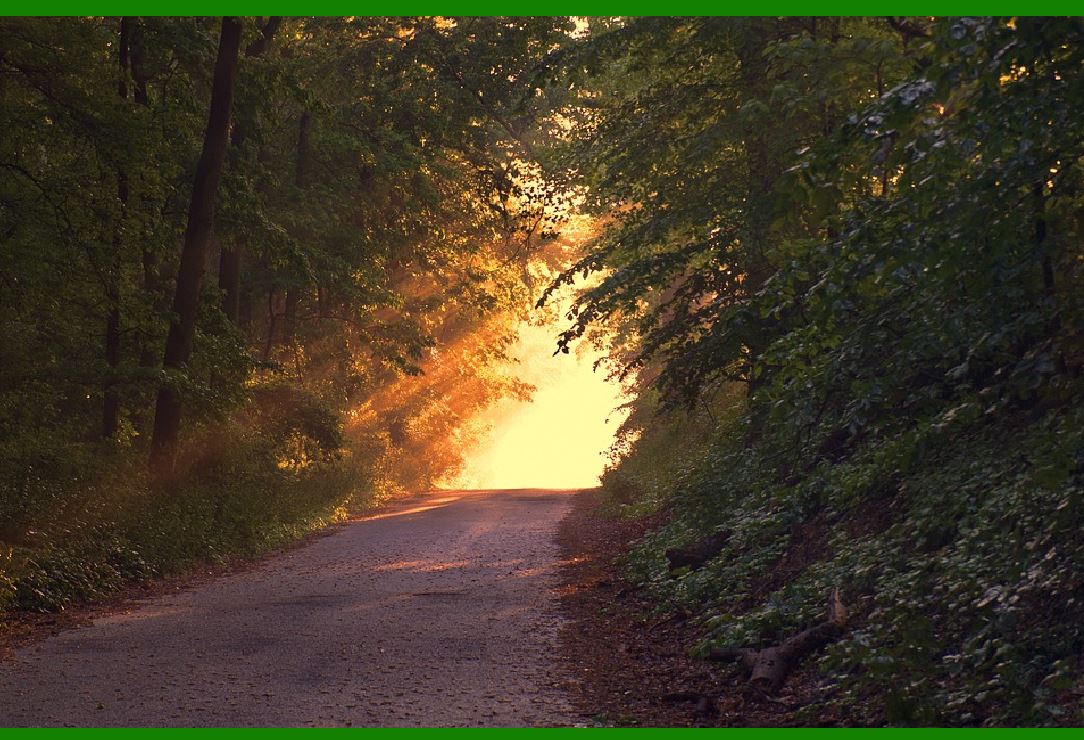
|
2. Privacy and Data Protection |
AI systems often rely on vast amounts of personal data to function effectively. This raises significant privacy and data protection concerns. The collection, storage, and processing of personal data by AI systems can lead to unauthorized access, data breaches, and misuse of sensitive information. |
2.1 Privacy Risks |
The privacy risks associated with AI include: |
Data Breaches: AI systems that handle large volumes of personal data are vulnerable to data breaches. Unauthorized access to sensitive information can have severe consequences for individuals, including identity theft and financial loss. |
Surveillance: AI technologies, such as facial recognition and location tracking, can be used for mass surveillance, infringing on individuals?privacy rights. This is particularly concerning in authoritarian regimes where such technologies can be used to monitor and control citizens. |
Informed Consent: Obtaining informed consent for data collection and use is challenging in the context of AI. Individuals may not fully understand how their data will be used or the potential risks involved. |
2.2 Mitigation Strategies |
To address privacy and data protection concerns, several measures can be implemented: |
Data Minimization: Limiting the amount of personal data collected and processed by AI systems can reduce privacy risks. This involves collecting only the data necessary for the intended purpose and anonymizing data where possible. |
Robust Security Measures: Implementing robust security measures, such as encryption and access controls, can help protect personal data from unauthorized access and breaches. |
Transparent Data Practices: Ensuring transparency in data collection and use practices can help build trust with individuals. This includes providing clear information about how data will be used and obtaining explicit consent. |
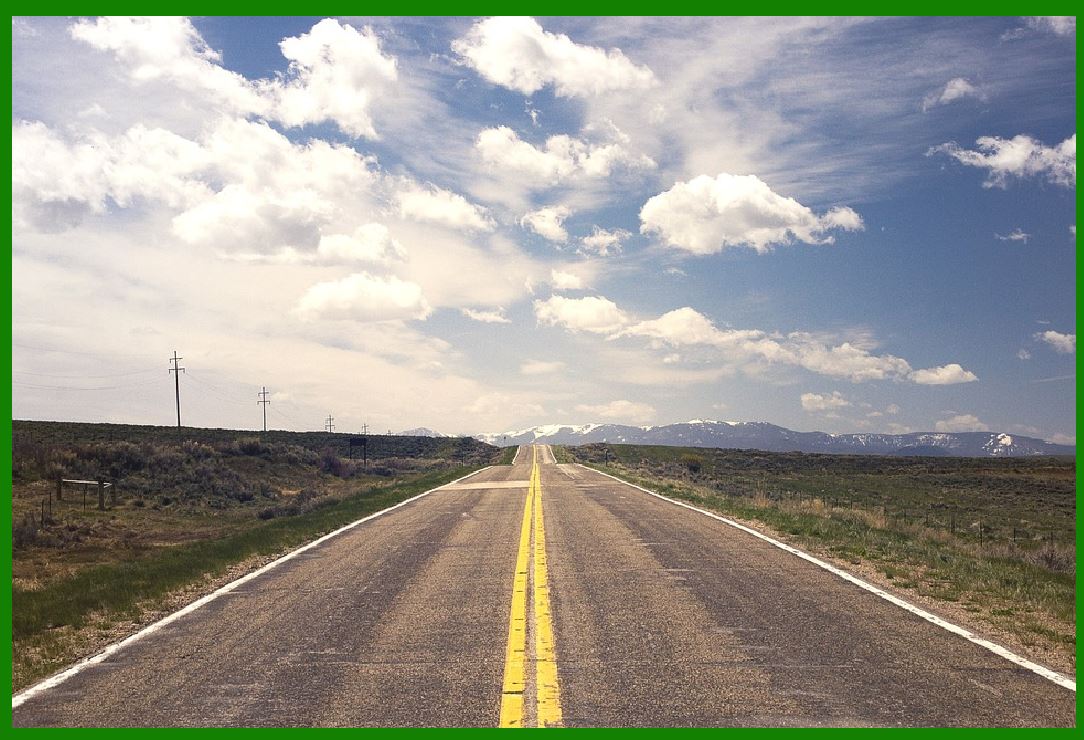
|
3. Accountability and Responsibility |
Determining accountability and responsibility for AI systems?actions is a complex ethical challenge. When AI systems make decisions or take actions that result in harm, it is often unclear who should be held accountable. This issue is particularly relevant in the context of autonomous systems, such as self-driving cars and automated decision-making systems. |
3.1 Challenges in Accountability |
The challenges in establishing accountability for AI systems include: |
Opacity of AI Systems: Many AI systems, particularly those based on deep learning, operate as “black boxes,?making it difficult to understand how they arrive at their decisions. This lack of transparency complicates the assignment of responsibility. |
Shared Responsibility: The development and deployment of AI systems often involve multiple stakeholders, including developers, manufacturers, and users. This shared responsibility can lead to ambiguity in determining who is accountable for the system actions. |
Legal and Regulatory Gaps: Existing legal and regulatory frameworks may not adequately address the unique challenges posed by AI. This can result in a lack of clear guidelines for assigning responsibility and accountability. |
3.2 Mitigation Strategies |
To address accountability and responsibility challenges, several approaches can be taken: |
Explainable AI: Developing AI systems that are transparent and explainable can help clarify how decisions are made and who is responsible. This involves creating models that provide clear and understandable explanations for their outputs. |
Clear Legal Frameworks: Establishing clear legal and regulatory frameworks for AI can help define accountability and responsibility. This includes creating guidelines for the development, deployment, and use of AI systems. |
Ethical Standards and Guidelines: Developing and adhering to ethical standards and guidelines for AI can help ensure responsible practices. This includes establishing codes of conduct and best practices for AI development and use. |
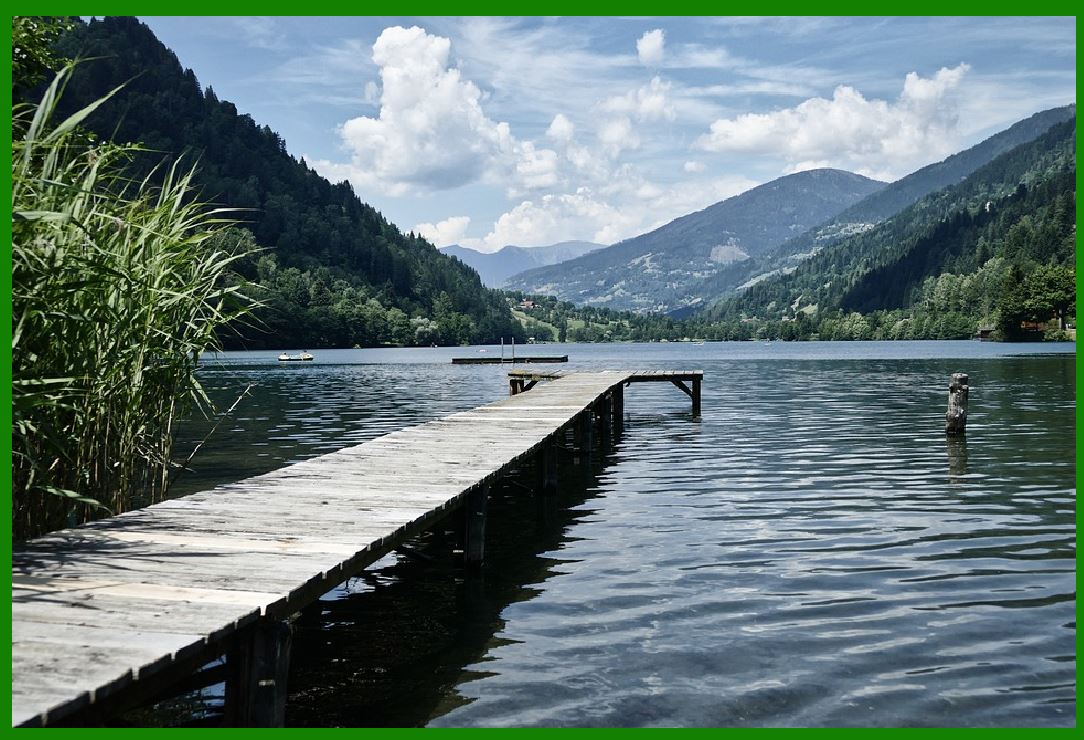
|
4. Job Displacement and Economic Impact |
The widespread adoption of AI technologies has the potential to significantly impact the job market and economy. While AI can increase efficiency and productivity, it can also lead to job displacement and economic inequality. |
4.1 Job Displacement |
AI and automation can replace certain job roles, leading to job displacement and unemployment. This is particularly concerning for low-skilled and repetitive jobs, which are more susceptible to automation. The impact of job displacement can be severe, leading to economic hardship and social instability. |
4.2 Economic Inequality |
The economic benefits of AI are not evenly distributed, leading to increased economic inequality. Companies and individuals with access to AI technologies can gain a competitive advantage, while those without access may be left behind. This can exacerbate existing economic disparities and create new forms of inequality. |
4.3 Mitigation Strategies |
To address job displacement and economic impact, several measures can be implemented: |
Reskilling and Upskilling: Investing in reskilling and upskilling programs can help workers adapt to the changing job market. This involves providing training and education to help individuals acquire new skills and transition to new roles. |
Social Safety Nets: Strengthening social safety nets, such as unemployment benefits and social security, can help mitigate the impact of job displacement. This includes providing financial support and assistance to those affected by automation. |
Inclusive Economic Policies: Implementing inclusive economic policies can help ensure that the benefits of AI are shared more equitably. This includes promoting access to AI technologies and supporting small and medium-sized enterprises. |
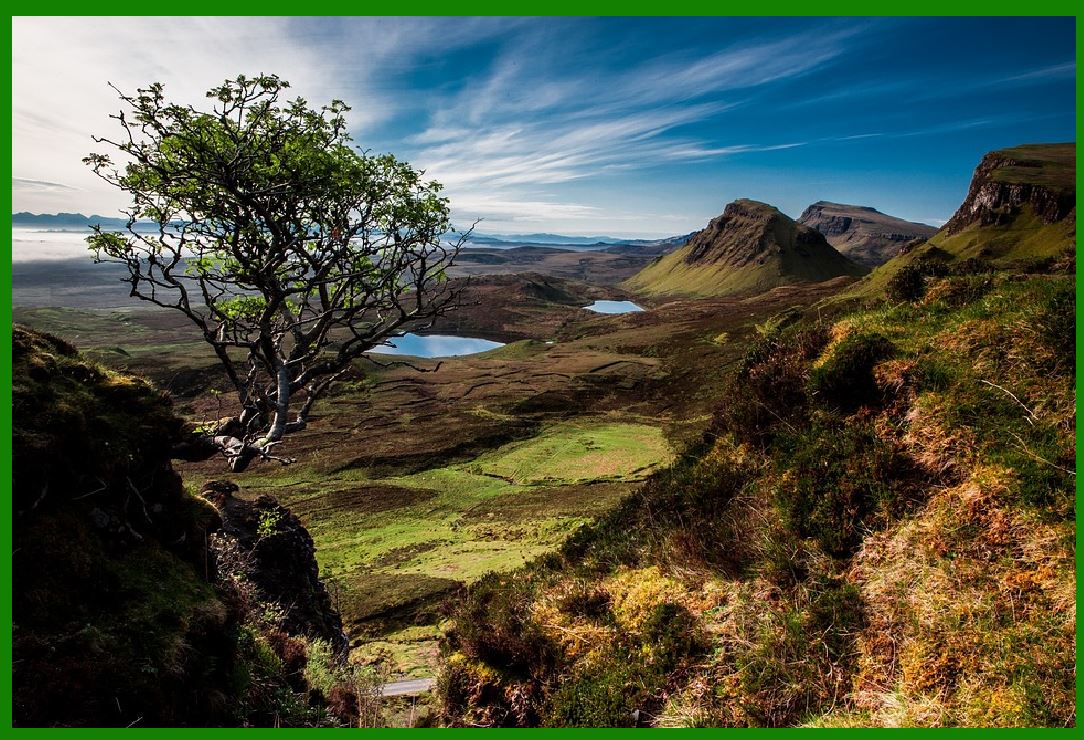
|
5. Ethical Use of AI in Decision-Making |
AI systems are increasingly being used to make decisions in various domains, including healthcare, finance, and criminal justice. The ethical use of AI in decision-making raises several concerns, including fairness, transparency, and accountability. |
5.1 Fairness in Decision-Making |
Ensuring fairness in AI decision-making is a significant ethical challenge. AI systems can produce biased and discriminatory outcomes if not properly designed and monitored. This is particularly concerning in areas such as criminal justice, where biased AI systems can lead to unfair treatment and wrongful convictions. |
5.2 Transparency and Explainability |
Transparency and explainability are crucial for the ethical use of AI in decision-making. Individuals affected by AI decisions have the right to understand how those decisions are made and to challenge them if necessary. However, many AI systems operate as “black boxes,?making it difficult to provide clear explanations for their decisions. |
5.3 Accountability in Decision-Making |
Establishing accountability for AI decisions is essential to ensure ethical use. This involves identifying who is responsible for the decisions made by AI systems and ensuring that they are held accountable for any negative outcomes. |
5.4 Mitigation Strategies |
To address ethical concerns in AI decision-making, several strategies can be employed: |
Fairness Audits: Conducting regular fairness audits can help identify and address biases in AI decision-making. This involves evaluating the outcomes of AI systems and making necessary adjustments to ensure fairness. |
Explainable AI: Developing explainable AI systems can help improve transparency and accountability. This involves creating models that provide clear and understandable explanations for their decisions. |
Ethical Guidelines: Establishing ethical guidelines for AI decision-making can help ensure responsible practices. This includes creating standards for fairness, transparency, and accountability. |
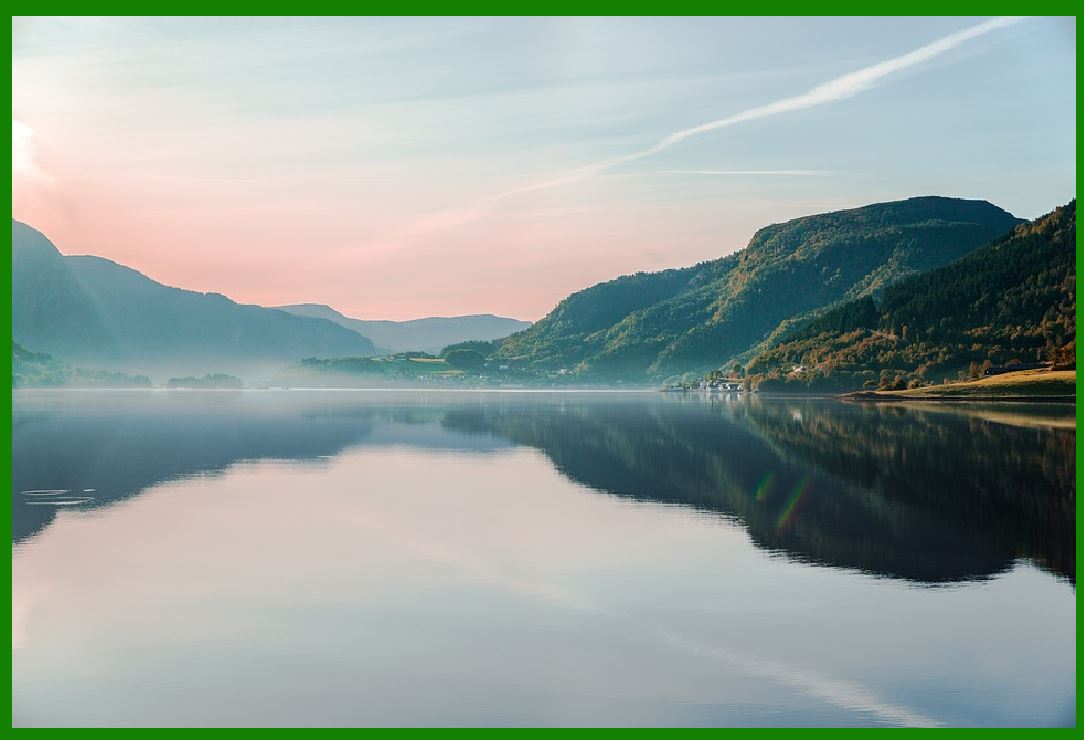
|
6. Environmental Impact |
The development and deployment of AI technologies have significant environmental implications. The energy consumption and carbon footprint of AI systems, particularly those based on deep learning, are substantial and raise concerns about sustainability. |
6.1 Energy Consumption |
AI systems, especially those that rely on large-scale data processing and deep learning, consume significant amounts of energy. The training of deep learning models requires substantial computational resources, leading to high energy consumption and carbon emissions. |
6.2 Resource Utilization |
The development and deployment of AI technologies also involve the use of various resources, including rare earth metals and other materials. The extraction and processing of these resources can have negative environmental impacts, including habitat destruction and pollution. |