1. Introduction to AI and Big Data |
1.1 Definition of Artificial Intelligence (AI) |
Artificial Intelligence (AI) refers to the simulation of human intelligence in machines that are programmed to think and learn like humans. These intelligent systems can perform tasks that typically require human intelligence, such as visual perception, speech recognition, decision-making, and language translation. AI can be categorized into three types: |
Narrow AI: Also known as Weak AI, it is designed to perform a narrow task (e.g., facial recognition or internet searches). |
General AI: Also known as Strong AI, it possesses the ability to perform any intellectual task that a human can do. |
Superintelligent AI: This is a hypothetical AI that surpasses human intelligence in all aspects. |
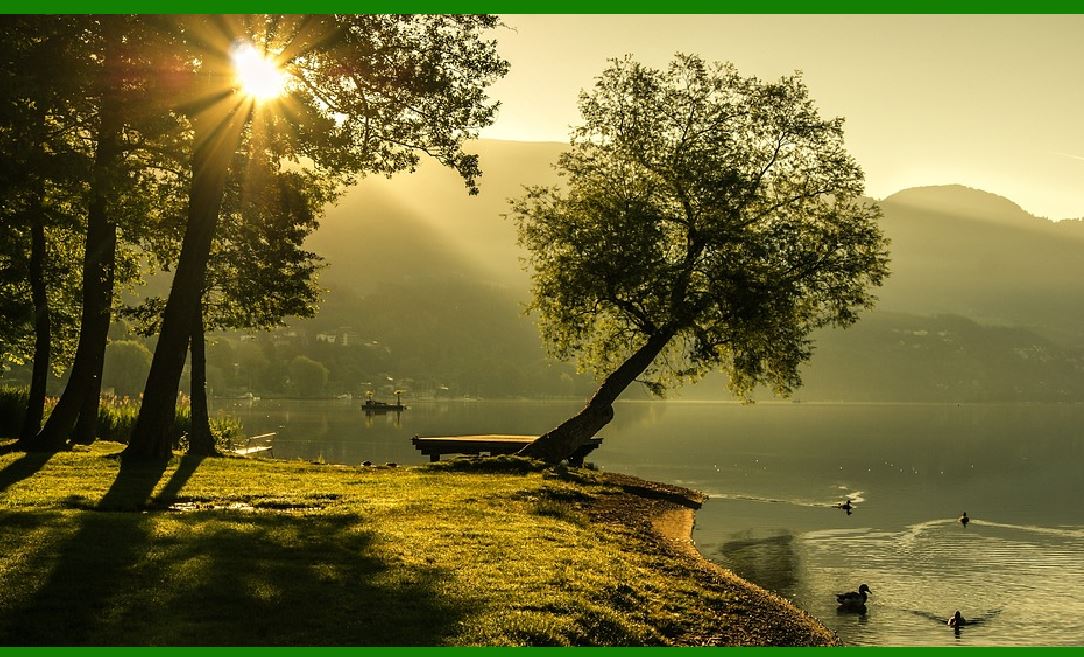
|
1.2 Definition of Big Data |
Big Data refers to extremely large datasets that may be analyzed computationally to reveal patterns, trends, and associations, especially relating to human behavior and interactions. The concept of Big Data encompasses the following characteristics, often referred to as the “Three Vs? |
Volume: The amount of data generated is vast, often measured in terabytes or petabytes. |
Velocity: The speed at which new data is generated and processed. |
Variety: The different types of data, including structured, semi-structured, and unstructured data. |
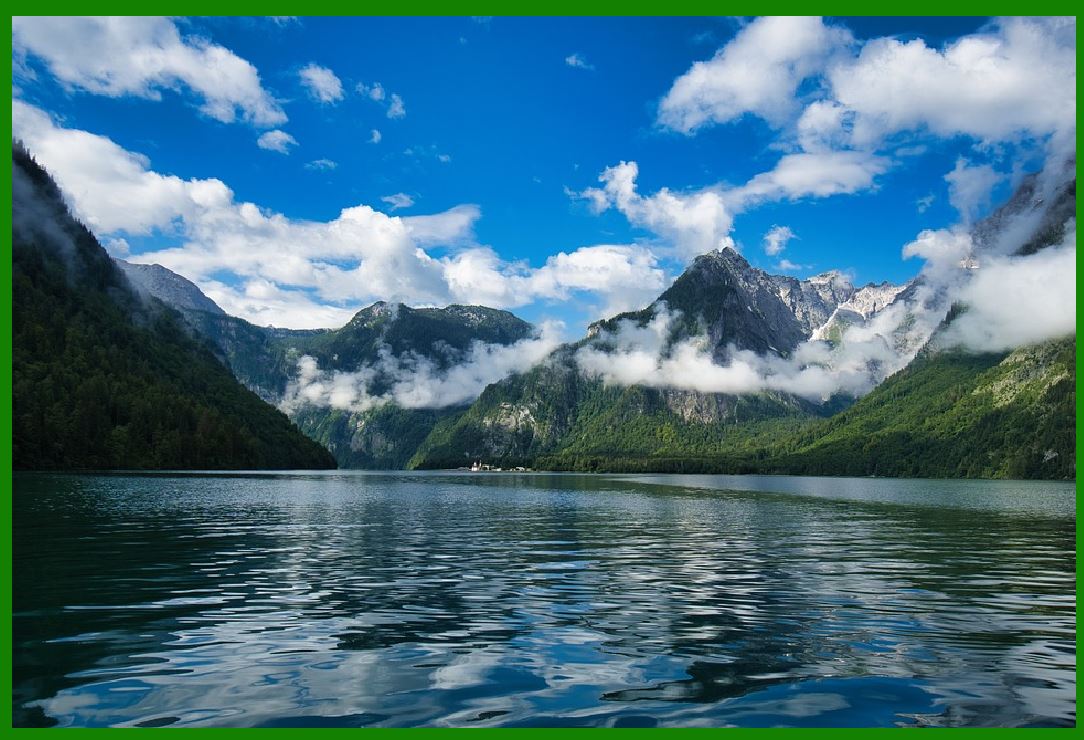
|
2. The Symbiotic Relationship Between AI and Big Data |
2.1 How AI Benefits from Big Data |
AI systems, particularly those based on machine learning and deep learning, require large amounts of data to train models effectively. The more data available, the better the AI can learn and make accurate predictions. Big Data provides the necessary volume, velocity, and variety of data that AI systems need to improve their performance. |
2.2 How Big Data Benefits from AI |
Conversely, AI technologies are essential for analyzing and making sense of Big Data. Traditional data processing methods are often inadequate for handling the complexity and scale of Big Data. AI algorithms can process and analyze large datasets more efficiently, uncovering insights that would be impossible to detect manually. |
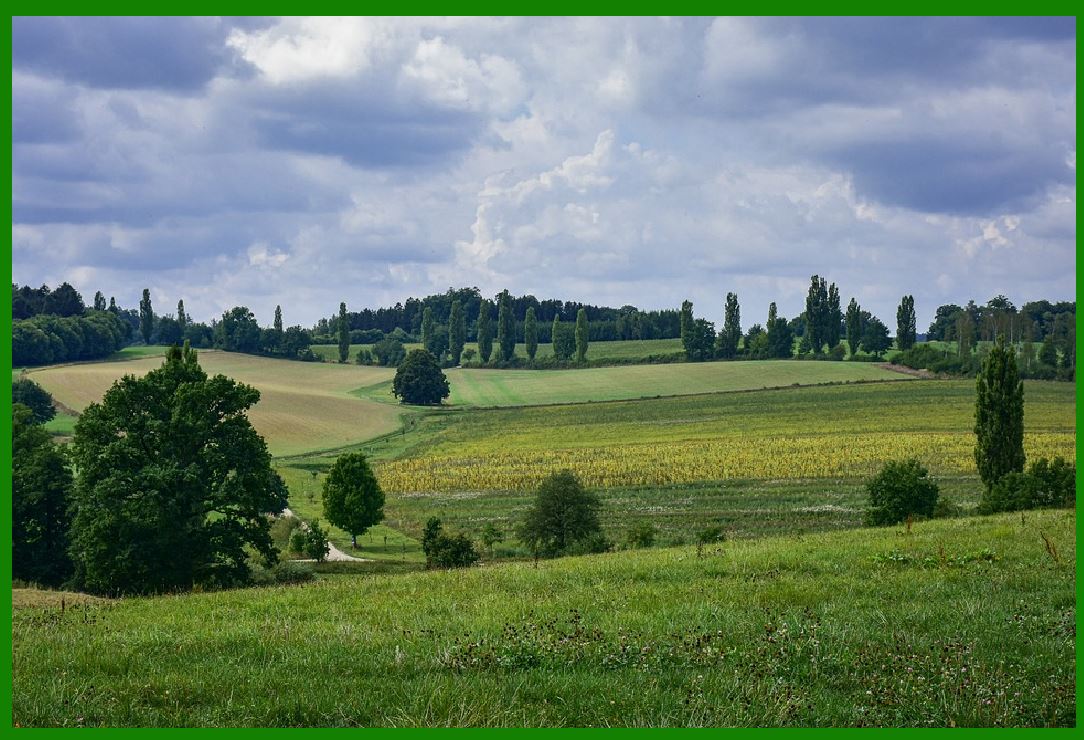
|
3. Applications of AI and Big Data |
3.1 Healthcare |
In healthcare, AI and Big Data are used to improve patient outcomes, enhance operational efficiency, and reduce costs. Examples include: |
Predictive Analytics: Using patient data to predict disease outbreaks or individual health risks. |
Personalized Medicine: Tailoring treatments based on individual genetic profiles and health data. |
Medical Imaging: AI algorithms analyze medical images to detect diseases such as cancer at an early stage. |
3.2 Finance |
In the financial sector, AI and Big Data are transforming how institutions operate and serve their customers. Applications include: |
Fraud Detection: Analyzing transaction data to identify and prevent fraudulent activities. |
Algorithmic Trading: Using AI to make high-frequency trading decisions based on market data. |
Customer Service: Implementing chatbots and virtual assistants to handle customer inquiries and transactions. |
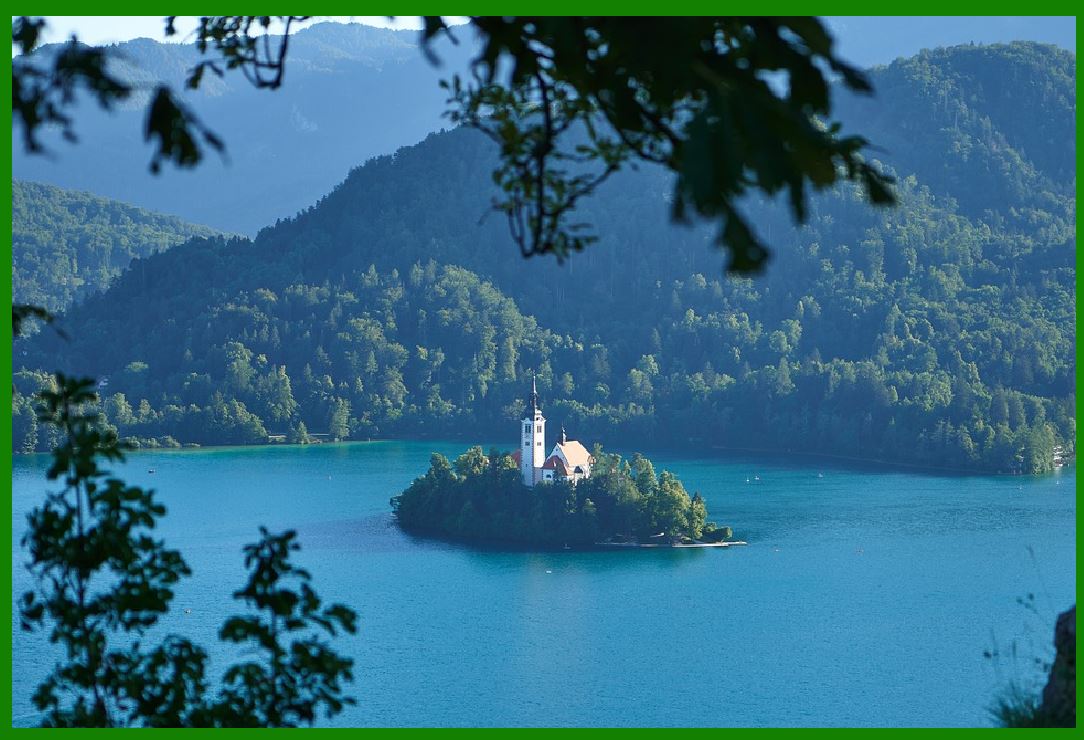
|
3.3 Retail |
Retailers leverage AI and Big Data to enhance customer experiences and optimize operations. Key applications include: |
Recommendation Systems: Analyzing customer data to provide personalized product recommendations. |
Inventory Management: Predicting demand and optimizing stock levels to reduce costs and improve availability. |
Customer Insights: Understanding customer behavior and preferences to tailor marketing strategies. |
3.4 Manufacturing |
In manufacturing, AI and Big Data are used to improve efficiency, quality, and safety. Examples include: |
Predictive Maintenance: Using sensor data to predict equipment failures and schedule maintenance proactively. |
Quality Control: Analyzing production data to detect defects and improve product quality. |
Supply Chain Optimization: Enhancing supply chain efficiency by analyzing data from various sources. |
3.5 Transportation |
AI and Big Data are revolutionizing the transportation industry by improving safety, efficiency, and customer satisfaction. Applications include: |
Autonomous Vehicles: Using AI to enable self-driving cars that can navigate and make decisions in real-time. |
Traffic Management: Analyzing traffic data to optimize traffic flow and reduce congestion. |
Logistics: Enhancing route planning and delivery efficiency through data analysis. |
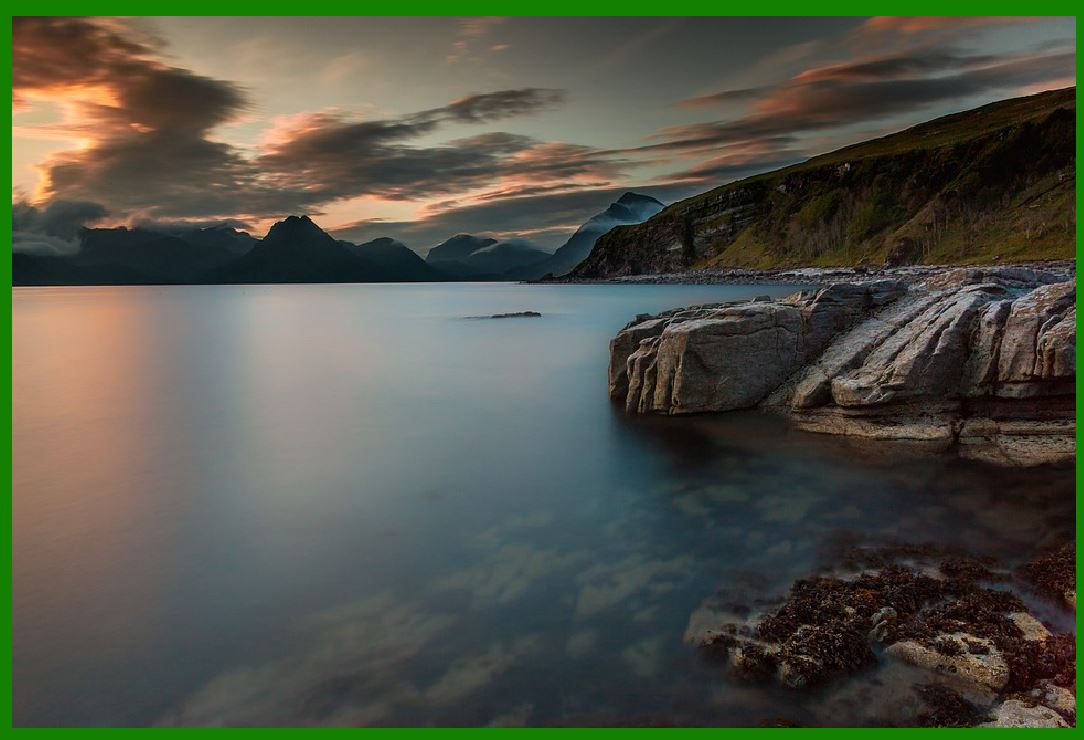
|
4. Challenges and Ethical Considerations |
4.1 Data Privacy and Security |
One of the primary concerns with AI and Big Data is data privacy and security. The collection and analysis of large amounts of personal data raise significant privacy issues. Ensuring that data is stored securely and used ethically is crucial to maintaining public trust. |
4.2 Bias and Fairness |
AI systems can inadvertently perpetuate biases present in the data they are trained on. This can lead to unfair outcomes, particularly in areas such as hiring, lending, and law enforcement. Ensuring that AI systems are fair and unbiased is a critical challenge. |
4.3 Transparency and Accountability |
AI systems often operate as “black boxes,?making decisions without providing clear explanations. This lack of transparency can be problematic, especially in high-stakes areas like healthcare and criminal justice. Ensuring that AI systems are transparent and accountable is essential for their responsible use. |
4.4 Technical Challenges |
The integration of AI and Big Data involves several technical challenges, including: |
Data Quality: Ensuring that the data used for analysis is accurate, complete, and relevant. |
Scalability: Developing systems that can handle the scale and complexity of Big Data. |
Interoperability: Ensuring that different systems and technologies can work together seamlessly. |
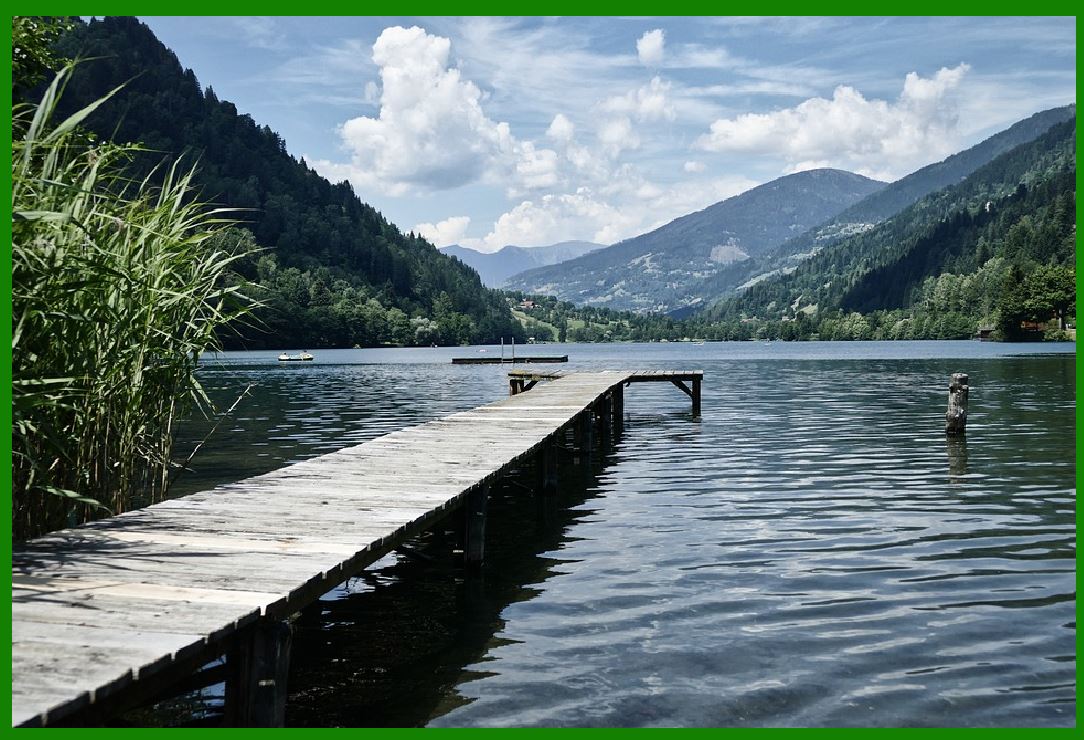
|
5. Future Trends in AI and Big Data |
5.1 Edge Computing |
Edge computing involves processing data closer to where it is generated, rather than relying on centralized data centers. This approach can reduce latency and improve the efficiency of AI and Big Data applications, particularly in areas like IoT and autonomous vehicles. |
5.2 Explainable AI |
Explainable AI (XAI) aims to make AI systems more transparent and understandable. By providing clear explanations for their decisions, XAI can help build trust and ensure that AI systems are used responsibly. |
5.3 Quantum Computing |
Quantum computing has the potential to revolutionize AI and Big Data by providing unprecedented computational power. This could enable the analysis of even larger datasets and the development of more advanced AI algorithms. |
5.4 AI Ethics and Governance |
As AI and Big Data continue to evolve, there will be an increasing focus on ethical considerations and governance. Developing frameworks and regulations to ensure the responsible use of these technologies will be crucial. |
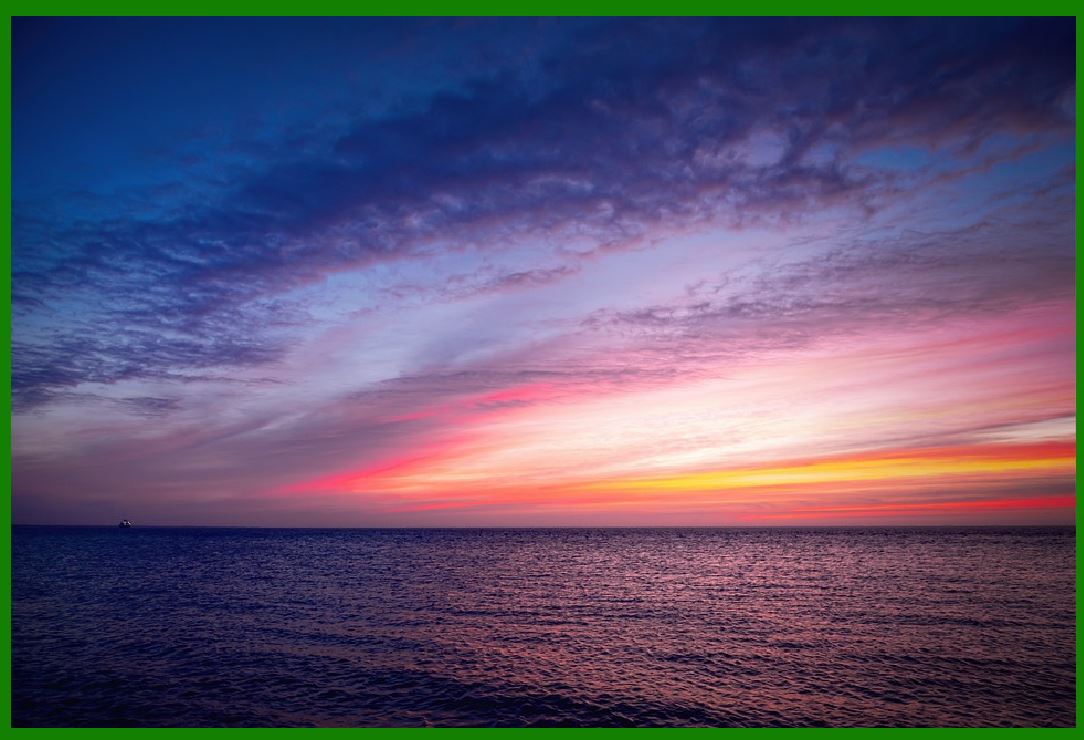
|
6. Conclusion |
AI and Big Data are two of the most transformative technologies of our time. Their symbiotic relationship enables the analysis of vast amounts of data, leading to insights and innovations that were previously unimaginable. While there are significant challenges and ethical considerations to address, the potential benefits of AI and Big Data are immense. As these technologies continue to evolve, they will undoubtedly play a crucial role in shaping the future of various industries and society as a whole. |